Tinder Swipes Right on AI to Help Stop Harassment
The dating app says its new machine-learning tool can help flag potentially offensive messages and encourage more users to report inappropriate…
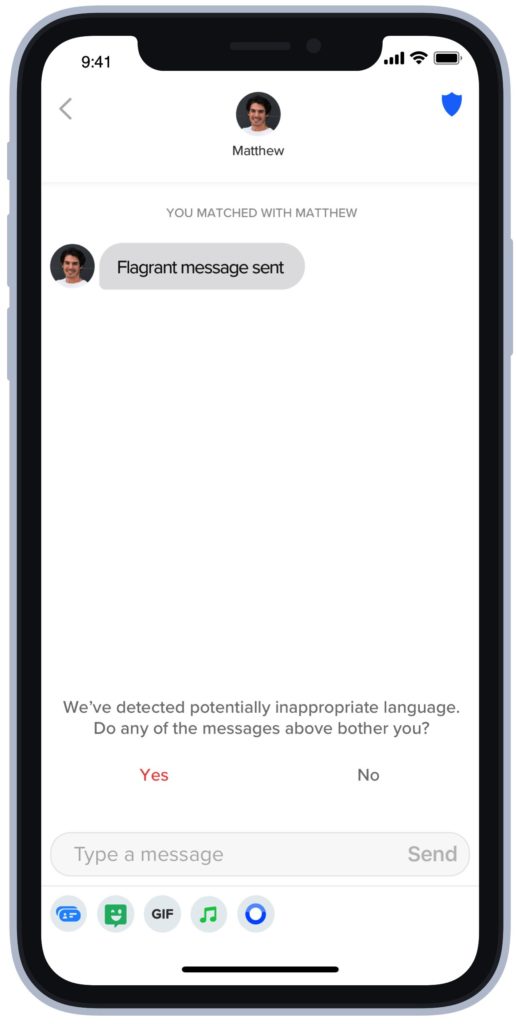
On Tinder, an opening line can go south pretty quickly. Conversations can easily devolve into negging, harassment, cruelty—or worse. And while there are plenty of Instagram accounts dedicated to exposing these “Tinder nightmares,” when the company looked at its numbers, it found that users reported only a fraction of behavior that violated its community standards.
Now, Tinder is turning to artificial intelligence to help people dealing with grossness in the DMs. The popular online dating app will use machine learning to automatically screen for potentially offensive messages. If a message gets flagged in the system, Tinder will ask its recipient: “Does this bother you?” If the answer is yes, Tinder will direct them to its report form. The new feature is available in 11 countries and nine languages currently, with plans to eventually expand to every language and country where the app is used.
Major social media platforms like Facebook and Google have enlisted AI for years to help flag and remove violating content. It’s a necessary tactic to moderate the millions of things posted every day. Lately, companies have also started using AI to stage more direct interventions with potentially toxic users. Instagram, for example, recently introduced a feature that detects bullying language and asks users, “Are you sure you want to post this?”
Tinder’s approach to trust and safety differs slightly because of the nature of the platform. The language that, in another context, might seem vulgar or offensive can be welcome in a dating context. “One person’s flirtation can very easily become another person’s offense, and context matters a lot,” says Rory Kozoll, Tinder’s head of trust and safety products.
That can make it difficult for an algorithm (or a human) to detect when someone crosses a line. Tinder approached the challenge by training its machine-learning model on a trove of messages that users had already reported as inappropriate. Based on that initial data set, the algorithm works to find keywords and patterns that suggest a new message might also be offensive. As it’s exposed to more DMs, in theory, it gets better at predicting which ones are harmful—and which ones are not.
The success of machine-learning models like this can be measured in two ways: recall, or how much the algorithm can catch; and precision, or how accurate it is at catching the right things. In Tinder’s case, where the context matters a lot, Kozoll says the algorithm has struggled with precision. Tinder tried coming up with a list of keywords to flag potentially inappropriate messages but found that it didn’t account for the ways certain words can mean different things—like a difference between a message that says, “You must be freezing your butt off in Chicago,” and another message that contains the phrase “your butt.”
Still, Tinder hopes to err on the side of asking if a message is bothersome, even if the answer is no. Kozoll says that the same message might be offensive to one person but totally innocuous to another—so it would rather surface anything that’s potentially problematic. (Plus, the algorithm can learn over time which messages are universally harmless from repeated no’s.) Ultimately, Kozoll says, Tinder’s goal is to be able to personalize the algorithm, so that each Tinder user will have “a model that is custom built to her tolerances and her preferences.”
Online dating in general—not just Tinder—can come with a lot of creepiness, especially for women. In a 2016 Consumers’ Research survey of dating app users, more than half of women reported experiencing harassment, compared to 20 percent of men. And studies have consistently found that women are more likely than men to face sexual harassment on any online platform. In a 2017 Pew survey, 21 percent of women aged 18 to 29 reported being sexually harassed online, versus 9 percent of men in the same age group.